5 Technical Challenges of AI in Convenience Stores
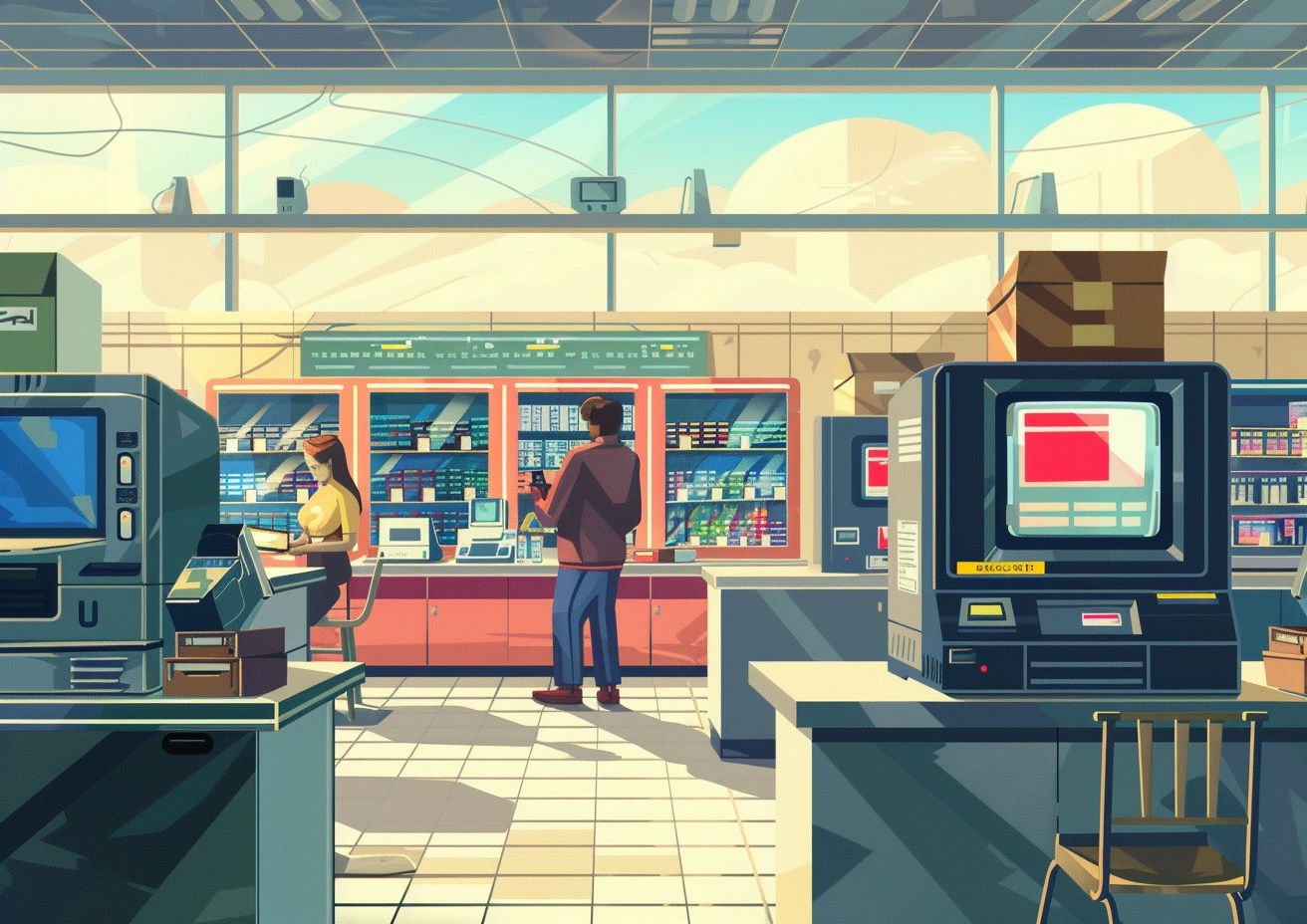
Implementing AI and computer vision in convenience stores to track customers' browsing and checkout activities represents a significant leap forward in retail innovation. These technologies promise to streamline operations, enhance the shopping experience, and offer insights into consumer behavior. However, technical difficulties abound, ranging from the accuracy of computer vision systems to the complexities of AI decision-making processes. This blog post delves into these challenges, exploring the intricacies of deploying AI and computer vision within the retail environment.
1. Accuracy of Computer Vision Systems
One of the primary technical challenges in using computer vision to track customers in convenience stores is ensuring system accuracy. Computer vision algorithms must correctly identify products, differentiate between similar items, and accurately monitor customer interactions with merchandise. This requires sophisticated image recognition capabilities, as items in a store can vary greatly in appearance due to packaging changes, lighting conditions, and placement angles.
For instance, accurately distinguishing between two nearly identical products with subtle differences in labeling or packaging can be problematic. The systems must be trained on a vast array of data to improve their recognition capabilities, a process that is both time-consuming and resource-intensive.
2. Real-Time Processing and Analysis
Another significant challenge is the need for real-time processing and analysis of the data captured by computer vision systems. Tracking customers and their interactions with products in real-time demands substantial computational resources and efficient algorithms capable of quick data processing.
The technology must process and analyze video feeds instantly to identify items picked up or returned by customers, requiring powerful servers and optimized software. The complexity increases with the number of customers in the store and the variety of items they interact with, making scalability a crucial concern.
3. Integration with Existing Systems
Integrating AI and computer vision technologies with existing retail management and inventory systems presents another layer of complexity. These systems must work seamlessly together to update inventory levels, process payments, and provide real-time insights into customer behavior.
This integration requires a robust IT infrastructure and can involve significant modifications to existing software and hardware. Retailers must ensure that the new technologies do not disrupt current operations while providing the intended benefits of enhanced customer tracking and inventory management.
4. Handling Dynamic Environments
Convenience stores are dynamic environments where products, displays, and lighting conditions can change frequently. AI and computer vision systems must be adaptable to these changes to maintain accuracy and effectiveness. This requires continuous learning and adjustment by the AI algorithms, a process known as machine learning.
However, machine learning models can be slow to adapt to new conditions, and their performance can degrade if not regularly updated with new data. Maintaining the accuracy of these systems over time in a constantly changing retail environment is a significant technical challenge.
Additionally, the scale of deployment affects not only the cost but also the complexity of the system. For instance, doubling the size of a store from the size of an average Amazon Go (around 3,000 square feet) to 6,000 square feet doesn't just double the cost; it also exponentially increases the number of sensors and cameras needed. Moreover, the hardware must be scalable and adaptable to different store sizes and layouts without necessitating custom solutions for each location
5. Customer Interactions and Behavior Analysis
Analyzing customer interactions and behavior through AI and computer vision involves deciphering a wide range of actions and intents. Customers may pick up items for inspection and return them, move items to different locations, or engage in behaviors that are difficult for AI systems to interpret accurately.
Developing algorithms that can understand the nuances of human behavior in a retail context is challenging. These systems must be trained on diverse datasets to recognize a wide variety of customer actions and intentions, a process that involves complex data analysis and algorithm development.
Conclusion
The implementation of AI and computer vision in convenience stores to track customer browsing and checkout activities is fraught with technical challenges. Ensuring the accuracy of computer vision systems, processing and analyzing data in real-time, integrating with existing retail systems, handling dynamic store environments, and analyzing complex customer behaviors are among the key difficulties faced by retailers.
Despite these challenges, advancements in AI and computer vision technologies continue to provide innovative solutions to these problems. With ongoing research and development, these technologies are becoming more sophisticated, promising to overcome current limitations and redefine the retail experience.
As the technology evolves, retailers will likely find more efficient ways to address these technical challenges, paving the way for broader adoption of AI and computer vision in the retail sector. For more detailed insights and examples of how AI and computer vision are being implemented in retail, exploring articles from reputable sources such as TechCrunch, Wired, and Retail Dive can provide further understanding and examples of current implementations and challenges faced.